The Future of Business: Harnessing the Power of Machine Learning
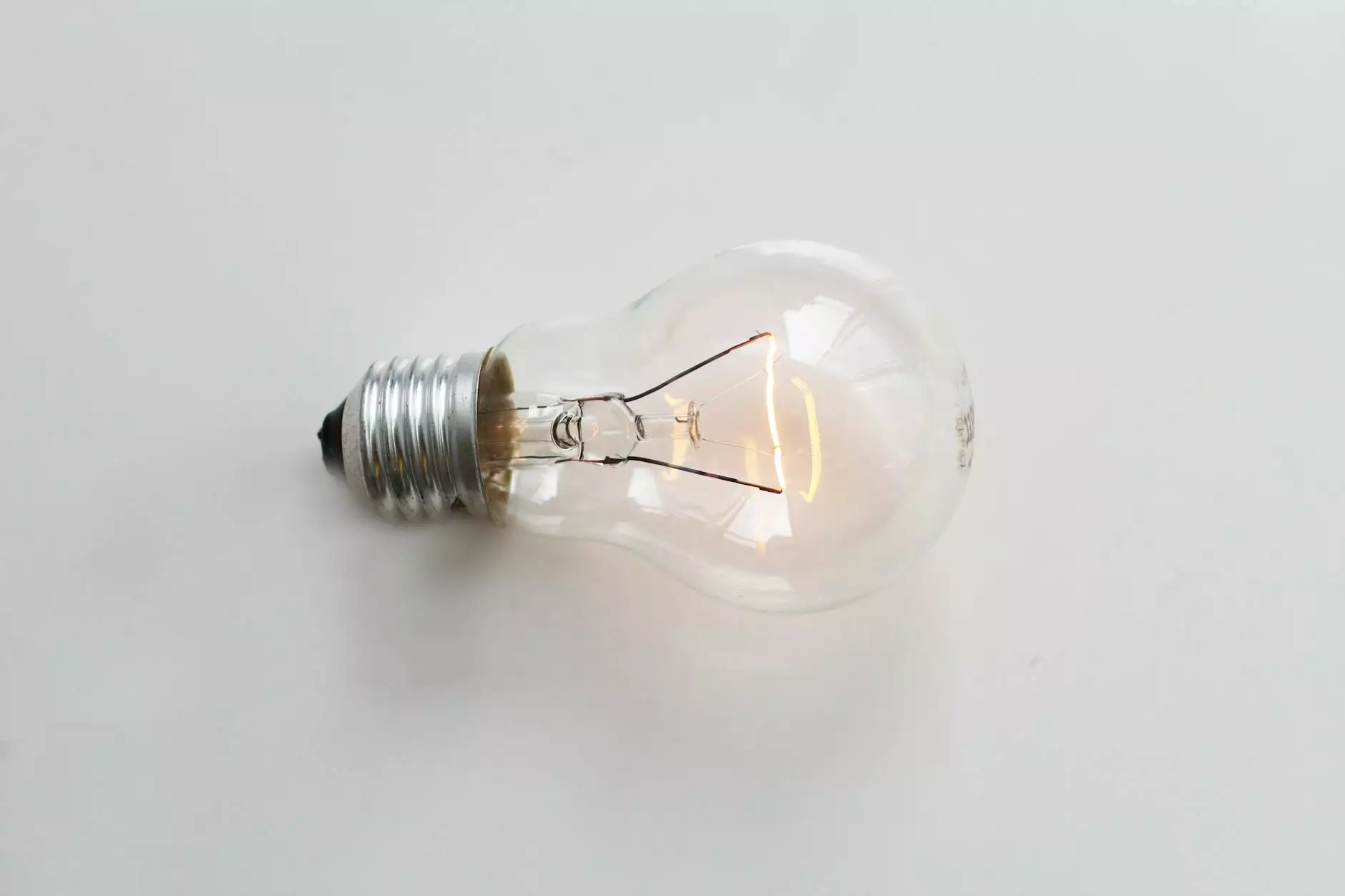
Machine learning (ML) is no longer a futuristic concept reserved for science fiction; it has become a transformative force reshaping the way businesses operate in 2023. Companies are increasingly turning to ML solutions to enhance their operational efficiencies, personalize customer experiences, and drive innovation. In this comprehensive guide, we will explore the various aspects of machine learning in business, highlighting its applications, benefits, challenges, and practical steps for implementation.
Understanding Machine Learning
Machine learning is a subset of artificial intelligence (AI) that focuses on building systems that learn from data, recognize patterns, and make decisions with minimal human intervention. Unlike traditional programming, where explicit instructions are provided, ML algorithms use statistical techniques to empower systems to improve at tasks over time. Here are the key components of machine learning:
- Algorithms: The mathematical models that process data to identify patterns and make predictions.
- Data: The foundation of ML; the more relevant data you feed into the system, the more accurate its predictions will be.
- Training: The process of feeding data into an algorithm so it can learn and improve its predictive capabilities.
- Validation: Ensuring the algorithm performs well on unseen data, indicating its generalizability.
- Deployment: Integrating the trained model into business operations for real-time decision-making.
Key Applications of Machine Learning in Business
The versatility of machine learning means its applications are widespread across various industries. Here are some key applications that can revolutionize business operations:
1. Predictive Analytics
Businesses can leverage machine learning to forecast trends and customer behavior. By analyzing historical data, companies can predict future outcomes, allowing for strategic planning and informed decision-making.
2. Customer Segmentation
With ML, businesses can analyze customer data to identify distinct segments, enabling personalized marketing efforts. This not only improves customer satisfaction but also increases conversion rates.
3. Fraud Detection
Financial institutions use machine learning algorithms to detect fraudulent activities by recognizing patterns associated with historical fraud cases. This proactive approach enhances security measures and protects assets.
4. Enhanced Customer Service
Chatbots and virtual assistants powered by machine learning can provide 24/7 customer support. These tools use natural language processing to understand customer inquiries and offer instantaneous responses, improving service and efficiency.
5. Supply Chain Optimization
ML can analyze data across the supply chain to identify inefficiencies and predict demand fluctuations. This leads to better inventory management, reduced costs, and improved service delivery.
Benefits of Incorporating Machine Learning into Your Business
Integrating machine learning into your enterprise can unlock a multitude of benefits:
- Increased Efficiency: Automating routine tasks and processes allows employees to focus on higher-value activities.
- Enhanced Decision Making: Data-driven insights generated through ML can enhance strategic decision-making across departments.
- Cost Reduction: With improved efficiency, businesses can lower operational costs and increase profitability.
- Innovation: ML opens new avenues for product development and service enhancement, keeping businesses competitive in changing markets.
- Improved Customer Experience: Personalized marketing and faster response times lead to higher customer satisfaction and loyalty.
Challenges in Implementing Machine Learning
While the benefits of machine learning are substantial, businesses must navigate several challenges:
- Data Privacy: Handling sensitive customer data raises ethical concerns and requires strict compliance with regulations like GDPR.
- Skills Gap: There is a shortage of professionals skilled in machine learning, making it challenging for companies to find the right talent.
- Integration with Existing Systems: Adapting ML solutions to existing infrastructure can be complex and resource-intensive.
- Model Bias: If not carefully constructed, ML models can perpetuate or even amplify biases present in the training data.
Steps to Successfully Implement Machine Learning in Business
To effectively integrate machine learning into your business model, follow these essential steps:
1. Define Your Goals
Identify specific business challenges that ML can address. Clear objectives help focus efforts and measure success accurately.
2. Invest in Data Infrastructure
Ensure that your business has the necessary data collection, storage, and processing capabilities. Quality data is the cornerstone of effective machine learning.
3. Assemble a Skilled Team
Build a team of data scientists, ML engineers, and domain experts who can collaborate to develop and implement ML solutions.
4. Choose the Right Tools
Select appropriate ML frameworks and tools that align with your business goals and data architecture. Some popular options include TensorFlow, PyTorch, and Scikit-learn.
5. Start Small
Begin with pilot projects that allow you to test and validate your ML models before scaling them across the organization.
6. Monitor and Adapt
Continuously monitor the performance of your ML systems and be prepared to iterate and improve based on results and feedback.
Real-World Examples of Machine Learning in Business
Numerous companies have successfully implemented machine learning solutions, showcasing the technology's potential:
1. Amazon
Amazon uses machine learning to provide personalized product recommendations, optimizing their sales and enhancing customer experience. Their recommendation engine accounts for a significant portion of total sales.
2. Netflix
Netflix utilizes machine learning algorithms for content recommendation, enhancing viewer engagement and satisfaction. By analyzing user preferences, Netflix tailors its content offerings to match subscribers' tastes.
3. Tesla
Tesla employs machine learning in its Autopilot feature, enabling advanced driver assistance systems. Continuous learning from data collected from vehicles improves the algorithms over time, enhancing safety and efficiency.
4. Google
Google's search algorithm employs machine learning to better understand user queries and deliver more relevant search results, significantly improving user experience.
The Future of Machine Learning in Business
As machine learning technology continues to advance, the potential for its application in business will expand further. Companies that proactively adapt to these innovations will likely lead their industries. Emerging trends to watch include:
- Greater Automation: Businesses will increasingly use ML to automate complex tasks, freeing humans for strategic roles.
- Improved Natural Language Processing: Enhanced NLP capabilities will lead to more sophisticated chatbots and virtual assistants.
- AI Ethics: Focus on responsible AI use, addressing biases and ensuring fair outcomes from ML systems.
- Real-Time Decision Making: The integration of real-time data analytics with ML models will enable instant decisions and actions.
Conclusion
In conclusion, machine learning represents a pivotal opportunity for businesses aiming to innovate and remain competitive in an increasingly digital landscape. By understanding its applications, benefits, and the steps for successful implementation, companies can harness the power of machine learning to unlock unprecedented growth. Embrace this technology today, and position your business for a successful future in the *machine learning-powered* era.
ml blog